Learning portal - Generalised propensity score matching (GPSM)
- Evaluation
- Evaluation
- Evaluation Methods
- Data Management
- Performance Monitoring and Evaluation Framework (PMEF)
- Monitoring and evaluation framework
Generalised propensity score matching (GPSM) is a refined evaluation tool used when almost all units participate in a programme, making traditional comparison methods inadequate. Focusing on the intensity of support given to each unit, it allows a detailed analysis of how different support levels impact outcomes.
Page contents
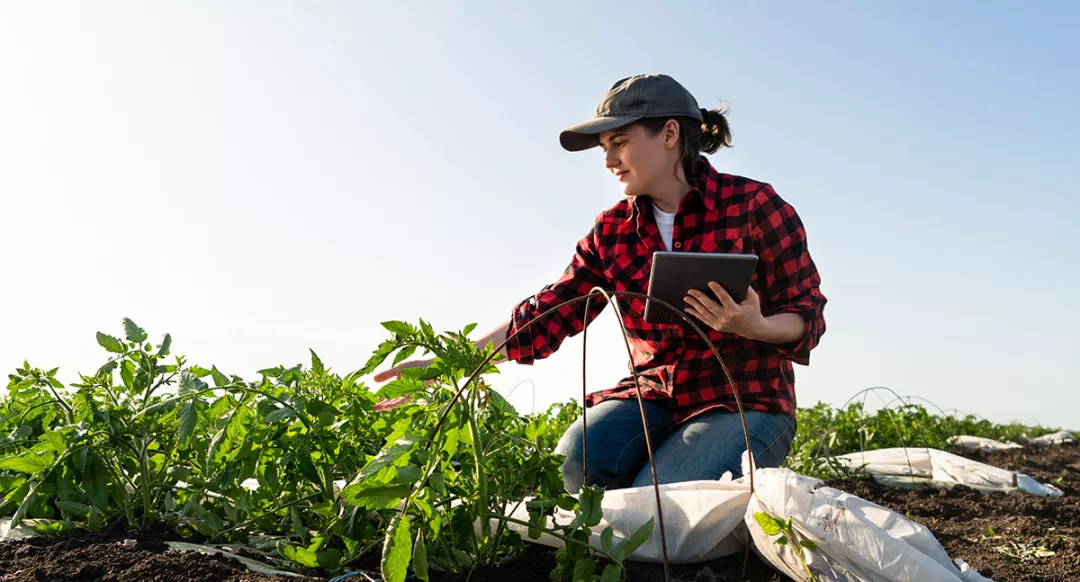
Basics
In a nutshell
The generalised propensity score matching (GPSM) method is a quasi-experimental evaluation methodology.
The technique is used when it is not possible to compare participants with non-participants because almost all units participate in the programme. In that case, the matching is not based on the probability of participating in the programme (as in the binary PSM) but on the probability of receiving a particular level of support from the programme (support intensity). Given explicit information on the intensity of support (e.g., financial flows into a public investment programme per farm, holding, community, or region), programme effects under this framework can be analysed using a dose-response function. A dose-response function explains the relationship between the intensity of the support provided (dose) and the outcome indicator (response).
The GPSM method allows not only the estimation of the average effect of public investment support on the selected outcome indicator but also the assessment of the marginal effects of the programmes or measures independent of the support intensity level obtained. Such disaggregated programme evaluation results cannot be obtained by employing traditional techniques e.g. the binary propensity score matching methodology, regression discontinuity design or any other techniques utilised in standard evaluation studies. As an extension of a binary PSM method, the GPSM method can also eliminate any bias associated with differences in the covariates included in an evaluation model.
Pros and cons
Advantages |
Disadvantages |
---|---|
|
When to use?
The GPSM approach is more general than PSM and can be used when all units received support from the given programme and the intensity of this support per unit is known (it also allows for the consideration of units that did not receive support i.e. level of intensity = 0). The method can be applied to assess programme effects at micro, regional or programme area levels. However, abundant data is required on the main characteristics and performance of programme beneficiaries (and non-beneficiaries) before and after the programme. An important assumption of this method is that the most important characteristics determining programme participation and performance can be observed.
Preconditions
- Abundant data on programme participants (and non-participants) before and after the programme.
- Ability to estimate the level of support intensity for each unit.
- Ability to calculate the value of the impact indicators for the different levels of intensity of the support.
- High quantitative skills (including econometrics) of the evaluator.
- Good understanding of conditions determining the probability of participation in the programme.
The technique can be applied to assess the effect of CAP support on the evolution of the values of the impact indicators listed in the following table.
RDP impact indicator | CAP Strategic Plan impact indicator |
---|---|
I.01 - Agricultural entrepreneurial income | I.2 - Evolution of agricultural income compared to the general economy |
I.02 - Agricultural factor income | I.3 - Evolution of agricultural income |
I.4 - Evolution of agricultural income level by type of farming (compared to the average in agriculture) | |
I.5 - Evolution of agricultural income in areas with natural constraints (compared to the average) | |
I.03 - Total factor productivity in agriculture | I.6 - Total factor productivity in agriculture |
I.07 - Emissions from agriculture |
I.10 - Greenhouse gas emissions from agriculture I.14 - Ammonia emissions from agriculture |
I.08 - Farmland bird index | I.19 - Farmland bird index |
I.09 - High nature value (HNV) farming | |
I.10 - Water abstraction in agriculture | I.17 - Water Exploitation Index Plus (WEI+) |
I.11 - Water quality |
I.15 - Gross nutrient balance on agricultural land I.16 - Nitrates in groundwater |
I.13 - Soil erosion by water | I.13 - Percentage of agricultural land in moderate and severe soil erosion |
I.14 - Rural employment rate | I.24 - Evolution of the employment rate in rural areas, including a gender breakdown |
I.15 - Degree of rural poverty | I.27 - Evolution of poverty index in rural areas |
I.16 - Rural GDP per capita | I.25 - Evolution of gross domestic product (GDP) per capita in rural areas |
Step-by-step
- Step 1 – Calculate the level of support for each unit. It is suggested to define the level of support not by using the absolute levels of support but rather the levels of support normalised by another variable, like the utilised agricultural area of the farmer or the area or population of a geographical region
- Step 2 – Divide the levels of support into pre-defined intervals.
- Step 3 – Collect data on all units and their significant characteristics (variables) at the start of the implementation period and at the time of the evaluation. Note that the variables included in the analysis must affect the outcome indicators.
- Step 4 – Apply appropriate techniques to create groups of units equally likely to receive a certain level of support (generalised propensity score) and belong to the same support interval (as defined in Step 2).
- Step 5 – Check the statistical similarity of the constructed groups (e.g. by performing statistical tests on covariates included in the analysis).
- Step 6 – Estimate the average value of the outcome indicator(s) for each support interval and the entire dose-response function.
- Step 7 – Perform a sensitivity analysis to assess the possible effects of unobservables on obtained results.
Main takeaway points
- GPSM allows for an in-depth evaluation of programmes where all units are participants, focusing on the intensity of support received.
- It provides a detailed analysis through the dose-response function, exploring how varying levels of support impact outcomes.
- GPSM offers unique insights, allowing for the estimation of average and marginal effects of support intensity.
- This method is particularly beneficial in evaluating complex programmes like those under the CAP, where interventions have varied intensity and impact on different objectives and themes.
- Its applicability depends heavily on the availability of comprehensive data and the evaluator’s quantitative skills.
Learning from practice
- Bia, M., & Mattei, A. (2007).
Application of the Generalized Propensity Score. Evaluation of public contributions to Piedmont enterprises - Hirano, K. and Imbens, G., (2004)
The Propensity score with continuous treatment, Missing data and Bayesian Method in Practice: Contributions by Donald Rubin Statistical Family - Imai, K. and van Dyk, D.A. (2004)
“Causal inference with general treatment regimes: Generalizing the propensity score”, Journal of the American Statistical Association, 99, 854-866 - Kluve, J., Schneider, H., Uhlendorff, A. and Zhao, Z. (2012)
Evaluating continuous training programmes by using the generalized propensity score. Journal of the Royal Statistical Society: Series A (Statistics in Society), 175: 587-617 - Michalek J., Ciaian P. and Kancs, d’A. (2014)
"Capitalisation of CAP Single Payment Scheme into Land Value: Generalised Propensity Score Evidence from the EU", Land Economics, 90(2), 260-289
Further reading
Assessing RDP Achievements and Impacts in 2019
- European Evaluation Network for Rural Development for the CAP (2014)
Capturing the success of your RDP: Guidelines for the ex post evaluation of 2007-2013 RDPs - Joint Research Centre, Institute for Prospective Technological Studies, Michalek, J. (2012)
Counterfactual impact evaluation of EU rural development programmes: propensity score matching methodology applied to selected EU Member States. Volume 2, A regional approach, Food and Agriculture Organization.