Learning portal - Propensity score matching-difference in differences (PSM-DiD) method
Propensity score matching-difference in differences (PSM-DiD), is an innovative technique that balances the comparison of programme participants and non-participants, before and after a programme's implementation. It offers a more accurate insight into the actual programme effects with minimal bias.
Page contents
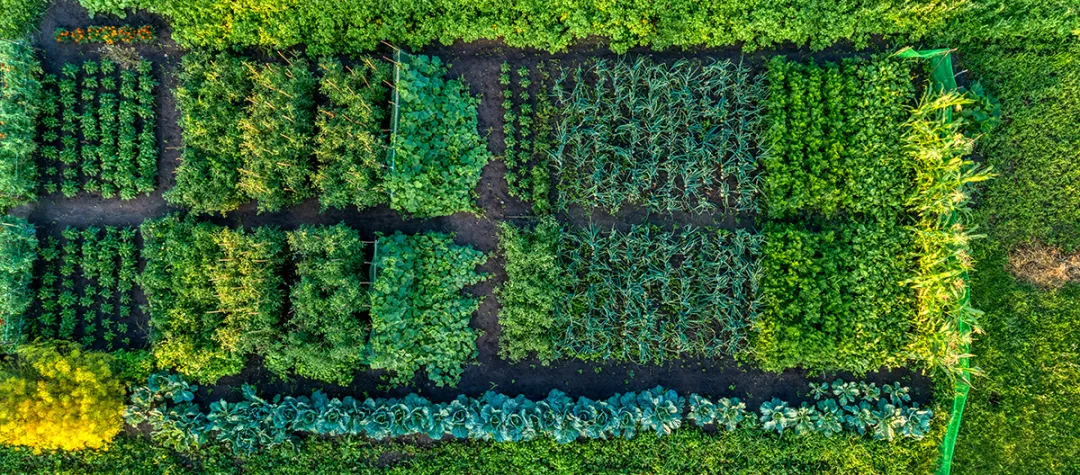
Basics
In a nutshell
The propensity score matching-difference in differences (PSM-DiD) method, also known as conditional DiD method, is a quasi-experimental evaluation methodology.
The technique is a highly applicable estimator when the outcome data on programme participants and non-participants is available before and after the programme's implementation. PSM-DiD measures the effect of the support by using the differences between comparable programme participants and non-participants before and after the implementation of a programme. In this method, programme participants are first matched with comparable non-participants, using the PSM technique. Then the net effect of the support is calculated as the difference in the average value of the impact indicator(s) between participants and non-participants, before and after implementing a programme.
A decisive advantage of the PSM-DiD estimator, compared to a conventional DiD estimator and the PSM technique applied separately, is that this method allows for better control of a selection bias in both observables (PSM) and unobservables (DiD). Selection bias occurs when participants in a programme are systemically different from non-participants. These systemic differences may be due to specific characteristics of participants and non-participants, which can be observed using variables available in the corresponding data sources (observables). For example, farms in certain sectors or with a specific economic or physical size may be more motivated to participate in the programme. This bias can be minimised by matching participants and non-participants, based on their observed characteristics, using the PSM technique (link to the PSM method). On the other hand, the systemic differences may come from characteristics which cannot be described using the available data (unobservables). For example, very similar participants and non-participants may differ in their internal motivation, which cannot be observed directly by any variable. To minimise this bias, the effect of unobserved characteristics is differenced out by subtracting the average value of the outcome indicator(s) of non-participants from the corresponding value of participants, before and after implementing a programme (DiD).
Thus, applying a conditional DiD estimator (PSM-DiD) to measure the effects of a programme may greatly improve evaluation findings, as it minimises the selection bias by constructing and comparing groups that are closer to what would have been constructed if participants and non-participants were randomly assigned.
Pros and cons
Advantages |
Disadvantages |
---|---|
|
|
When to use?
The method can be applied to assess programme effects at both micro, regional or programme area levels. However, it requires abundant data on the main characteristics and economic performance of programme beneficiaries and non-beneficiaries before and after a programme. An important assumption for this method is that the most important characteristics determining programme participation and performance can be observed.
Preconditions
- Abundant data on programme participants and non-participants prior to and after a programme (panel data).
- High quantitative skills of the evaluator.
- Good understanding of conditions determining the probability of participation in a programme.
The technique can be applied to assess the effect of CAP support on the evolution of the values of the impact indicators listed in the following table.
RDP impact indicator | CAP Strategic Plan impact indicator |
---|---|
I.01 - Agricultural entrepreneurial income | I.2 - Evolution of agricultural income compared to the general economy |
I.02 - Agricultural factor income | I.3 - Evolution of agricultural income |
I.03 - Total factor productivity in agriculture | I.6 - Total factor productivity in agriculture |
I.07 - Emissions from agriculture |
I.10 - Greenhouse gas emissions from agriculture I.14 - Ammonia emissions from agriculture |
I.08 - Farmland bird index | I.19 - Farmland bird index |
I.09 - High nature value (HNV) farming | |
I.10 - Water abstraction in agriculture | I.17 - Water Exploitation Index Plus (WEI+) |
I.11 - Water quality |
I.15 - Gross nutrient balance on agricultural land I.16 - Nitrates in groundwater |
I.13 - Soil erosion by water | I.13 - Percentage of agricultural land in moderate and severe soil erosion |
I.14 - Rural employment rate | I.24 - Evolution of the employment rate in rural areas, including a gender breakdown |
I.15 - Degree of rural poverty | I.27 - Evolution of poverty index in rural areas |
I.16 - Rural GDP per capita | I.25 - Evolution of gross domestic product (GDP) per capita in rural areas |
Specifically for the Farmland Bird Index, an assessment approach conducted at the field/plot scale level (micro level) can be realised by using the Common Birds Monitoring Programme if sufficient data is available. This can be achieved by applying the PSM-DiD technique to match beneficiaries and non-beneficiaries and then comparing the average CAP support effect on biodiversity in each group before and after the implementation of a programme. At the macro level, PSM is also recommended to net out the CAP support effect on biodiversity at the level of quadrants used to observe the farmland birds’ populations under the Common Birds Monitoring Programme. The quadrants can be used as functional units for the Farmland Bird Index and then calculated by bio-geographical areas (different agricultural habitats) or at the regional level based on geo-referenced data.
Regarding indicators measuring employment, GDP per capita and poverty rate in rural areas, the beneficiaries’ and control groups can be constructed based on supported and non-supported geographical regions, ideally LAU 2 as defined by the Eurostat urban-rural typology. In this case, access to a comprehensive source of statistical data and information about characteristics of geographical regions before and after the implementation of the programme is required.
Step-by-step
- Step 1 – Find a sample of beneficiaries (e.g. farms, farmers, non-farming enterprises, communities, areas, regions etc.) in an available database (e.g. FADN) and use the monitoring and evaluation electronic system (e.g. the electronic information system of Article 70, Regulation (EU) 1305/2013, or the one of Article 130, Regulatio (EU) 2021/2115) as a reference point.
- Step 2 – In the same database (e.g. FADN), select all relevant units that did not receive support or received an arbitrary low level of support (non-beneficiaries) in the same period. In the latter case of arbitrary low level of support, it might be better to define the low level by not using absolute levels of support but rather the levels of support normalised by another variable, like the utilised agricultural area of the farmer or the area or population of a geographical region.
- Step 3 – In a group of non-beneficiaries, identify those units which could not fulfil the programme eligibility conditions (due to high income, size, location, etc.) and remove them from the analysis.
- Step 4 – Collect data for all units in both groups (beneficiaries and non-beneficiaries) on their significant characteristics (variables) at the start of an implementation period. Note that the variables included in the analysis should affect both the selection of a unit and the indicators computed at the micro-level (common and additional impact indicators). Some of the proposed variables (used as important control variables) can be:
- the level of support received by a given unit during previous programming periods; and/or
- the level of support received by a given unit from other public sources (e.g. EU structural funds, Pillar I) in the analysed period.
- Step 5 – Apply appropriate techniques to identify a suitable control group from the sample of non-beneficiaries (see Steps 2-3), the members of which have the same propensity to participate in a programme (some of the non-beneficiaries and/or beneficiaries will be dropped from the analysis due to a lack of adequate control units).
- Step 6 – Check statistically the similarity of both groups prior to receiving support from a programme (e.g. by performing statistical tests on covariates included in the analysis). The average value of a unit in the beneficiary group should not significantly differ from the respective unit in the control group. Once the beneficiaries’ group and the control group have been constructed, the net effect of the support can be estimated through the subsequent steps.
- Step 7 – Compute the average value of outcome indicator(s) for the group of beneficiaries and the control group before the support and when the evaluation is carried out.
- Step 8 – Calculate the difference in the average value of the outcome indicator(s) for the beneficiaries’ group before the support and at the time of the evaluation (first difference).
- Step 9 – Calculate the difference in the average value of the outcome indicator(s) for the non-beneficiaries group before the support and at the time of the evaluation (second difference).
- Step 10 – Calculate the net effect of CAP support by subtracting the second difference (Step 9) from the first difference (Step 8).
- Step 11 – Perform a sensitivity analysis (e.g. Rosenbaum bounding approach) to assess the possible effects of unobservables on obtained results.
- Step 12 – Aggregate the findings and calculate the CAP support effects on the analysed impact indicators at the macro and programming area levels. In this step, the evaluator should calculate net direct effects of the CAP support on the impact indicators at the programme area level by applying extrapolation techniques (i.e. by multiplying average micro-results computed at a micro level by the number of beneficiaries/non-beneficiaries).
Main takeaway points
- PSM-DiD is very effective in controlling both observable and unobservable selection bias.
- The method involves matching participants with similar non-participants, and then measuring changes in impact indicators over time.
- It is most effective in situations with comprehensive data and requires an evaluator with a strong quantitative background.
- PSM-DiD offers detailed insights into the effectiveness and efficiency of interventions.
- The success of PSM-DiD depends on the depth of the available data and the methodology used for matching and comparison.
Learning from practice
Assessing Synergies in the Slovak RDP 2014-2020
- Caliendo, M., & Kopeinig, S. (2008)
Some Practical Guidance for the Implementation of Propensity Score Matching. Journal of Economic Surveys, 22(1), 31-72
Further reading
Assessing RDP Achievements and Impacts in 2019
- European Evaluation Network for Rural Development for the CAP (2014)
Capturing the success of your RDP: Guidelines for the ex post evaluation of 2007-2013 RDPs