Learning portal - Instrumental Variables (IV) method
- Evaluation
- Evaluation
- Evaluation Methods
- Data Management
- Performance Monitoring and Evaluation Framework (PMEF)
- Monitoring and evaluation framework
The instrument variables (IV) method is a powerful technique for identifying causal effects. Its main feature is using instruments to isolate a programme's impact when data is scarce or confounded, providing more accurate evaluations.
Page contents
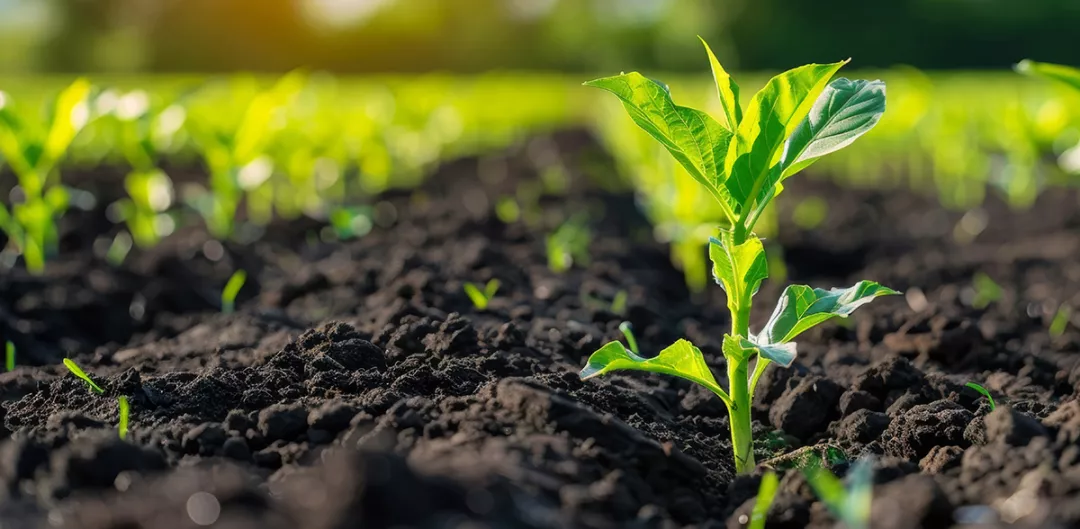
Basics
In a nutshell
The instrumental variable method (IV) deals with cases where the decision to participate in a given programme is mostly due to characteristics that cannot be observed and data unavailable on beneficiaries and non-beneficiaries before implementing a programme. In these cases, an instrumental variable may help identify the causal effect and must have two characteristics:
- it must be related to programme participation, i.e. it affects the decision to apply for a programme; and
- it cannot be correlated with an outcome indicator (except through programme participation) or unobserved variables correlated with an outcome indicator.
The choice of instrument is the most crucial step in implementing this method and should be carefully informed by economic intuition or theory.
Common sources of instruments may include:
- Policy geographical variation. For instance, if, for exogenous reasons, the policy is only implemented in some regions and not in others, only part of the population will be exposed to the policy.
- Exogenous shocks affecting the timing of policy implementation. For instance, if, for exogenous reasons, the policy implementation was delayed in one region or for some population group.
- Policy eligibility rules. For example, if the policy is designed so that some units are eligible while others are not, parallel with the regression discontinuity design method.
Pros and cons
Advantages |
Disadvantages |
---|---|
|
|
When to use?
This technique can be used when problems in the available data affect the estimates' internal validity. These problems may include:
- confounding variables, that is, variables that may affect the changes in the value of outcome indicators but cannot be controlled due to the lack of data;
- simultaneity, that is, when (some of) the observed variables that affect the changes in the value of outcome indicators are also affected by the outcome indicators;
- reverse causality, that is, when (some of) the observed variables, instead of affecting the changes in the value of outcome indicators, are themselves affected by these indicators.
IV might be a solution in such cases where there is limited availability of data to apply more robust estimators. The IV approach can assess programme effects when an evaluator can find and select good instruments (explicit variables) satisfying the conditions mentioned above. Estimating programme effects requires econometric estimation (2-stage least squares, panel estimates with fixed effects, etc.) of a functional form with programme participation as an endogenous variable. The IV approach also involves testing the importance of endogeneity (that is, the correlation between the instrumental variable(s) and the error term) and requires abundant data (cross-sectional or panel).
Preconditions
- Available cross-sectional or panel data on programme participants and non-participants.
- Ability to identify appropriate instruments.
- High quantitative skills (incl. econometrics) of the evaluator.
- Good understanding of conditions determining the probability of participation in the programme.
The technique can be applied to assess the effect of CAP support on the evolution of the values of the impact indicators listed in the following table.
RDP impact indicator | CAP Strategic Plan impact indicator |
---|---|
I.07 - Emissions from agriculture |
I.10 - Greenhouse gas emissions from agriculture I.14 - Ammonia emissions from agriculture |
I.10 - Water abstraction in agriculture | I.17 - Water Exploitation Index Plus (WEI+) |
I.11 - Water quality |
I.15 - Gross nutrient balance on agricultural land I.16 - Nitrates in groundwater |
I.13 - Soil erosion by water | I.13 - Percentage of agricultural land in moderate and severe soil erosion |
I.14 - Rural employment rate | I.24 - Evolution of the employment rate in rural areas, including a gender breakdown |
I.15 - Degree of rural poverty | I.27 - Evolution of poverty index in rural areas |
I.16 - Rural GDP per capita | I.25 - Evolution of gross domestic product (GDP) per capita in rural areas |
Step by step
- Step 1 – Choose the instrumental variables: The choice of the instrument is crucial to ensure estimating the causal treatment effect. In general, detailed information on how a policy was targeted and implemented may reveal sources of exogenous variation that could be used as instrumental variables.
- Step 2 – Estimate the effect: Usually, this involves two stages. In the first stage, participation in a programme is estimated using the instrumental variable and other exogenous covariates. In the second stage, outcome indicators are estimated using an independent variable of the estimated participation calculated in the first stage.
- Step 3 – Assess the relation between an instrument and participation in a programme: This is a test for the first assumption of an instrumental variables method, stating that an instrument should be related to participation in a programme. This can be done by analysing the first stage described in Step 2 and assessing the strength of the relationship between participation in a programme and instrumental variables. If an instrument is weak in predicting participation in a programme, the estimation of the outcome indicator is seriously affected.
- Step 4 – Discuss the exclusion restriction: Even though the assumption that an instrument is not related to the error term is not directly testable, the evaluator should discuss extensively why it is believed that the instrumental variable and the error term are not correlated, relying, for instance, on economic theory and intuition.
Main takeaway points
- An instrumental variable helps isolate the causal effect in cases of unobservable influencing factors.
- The IV method addresses data limitations and confounding factors in programme evaluation.
- Common sources for instrumental variables include policy variations and eligibility rules.
- Key assumptions: the instrument must relate to programme participation and not be correlated with the outcome indicator, barring through a programme.
- The IV method is good at identifying causal relationships in complex evaluation scenarios.
Learning from practice
- Olagunju, K.O., Patton, M., Feng, S. (2020)
Estimating the Impact of Decoupled Payments on Farm Production in Northern Ireland: An Instrumental Variable Fixed Effect Approach. Sustainability, 12(8), 3222 - Petrick, Martin; Zier, Patrick (2012)
Common Agricultural Policy effects on dynamic labour use in agriculture, Food Policy, ISSN 0306-9192, Elsevier, Amsterdam, Vol. 37, Iss. 6, 671-678
Further reading
- European Evaluation Network for Rural Development for the CAP (2014)
Capturing the success of your RDP: Guidelines for the ex post evaluation of 2007-2013 RDPs - Joint Research Centre, Institute for the Protection and Security of the Citizen, Loi, M., Rodrigues, M. (2012)
A note on the impact evaluation of public policies: the counterfactual analysis, Publications Office